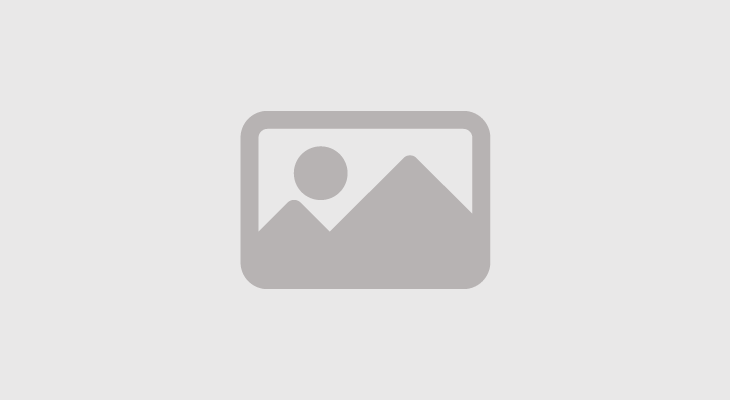
Jane Reed Highlights the Growing Dependence of NLP to Comply with the Regulatory Guidelines
Shots:
-
In an invigorating conversation with PharmaShots, Jane Reed discusses the transition from document-driven to data-driven decision-making in the pharmaceutical industry
-
Jane sheds light on the increasing dependence on AI and ML in regulatory affairs and how it helps pharma companies mitigate errors, discrepancies, and non-compliance
-
Jane highlights the benefits of NLP in ensuring regulatory compliance by citing two engaging case studies
Saurabh: Can we talk about what are the key trends in terms of regulatory challenges (common) faced by pharma companies?
Jane: We can think about three key trends. Across regulatory affairs, as with many aspects of pharmaceutical drug development, capitalizing on innovations in artificial intelligence and machine learning is one of the top ones. There’s also the impact of how to leverage real-world data and the increasing role of patient voice in regulatory decision-making. Also, pharma companies need to stay abreast with the frequently changing nature of regulatory guidelines and regulatory data.
Delving a little deeper into the last one; as an example, it’s essential for pharma companies to keep their drug labels up to date. If there is a change in regulatory guidance around a particular ingredient, or if you’re marketing a generic drug and there is a label change by a company that developed the drug, then you must change your label within set timeframes. So not only do you need to keep up to date with what the regulators are saying, you have to keep up to date with what your competitors are saying, as well. Much of the information you need – from regulatory guidelines, regulatory labels, regulatory documents, and drug monographs – is buried in text-based documents. For pharma companies, it is a substantial burden to understand what the latest compliances are and then make sure that you're in line. And this links to the first trend – bringing AI/ML into regulatory affairs, to help with the digital transformation from document-driven to data-driven decision-making.
Saurabh: How does the heavily regulated nature of the pharmaceutical industry pose challenges for companies in terms of regulatory compliance?
Jane: Pharma companies face a number of challenges related to regulatory compliance. One of the key issues is, simply understanding what all the new changes in regulations are. That can be complex because, while there is increasing global harmonization, there are still often different regulatory changes in different regions. The FDA may mandate something that is different from what EMA regulators demand, for example.
Another challenge involves timeliness in responding to these changes. As guidance is published by regulators and health agencies, pharma companies need to respond in a timely fashion. Some changes will trigger impact assessments which can be time-consuming and burdensome. Often, there are time limitations to responding. Knowing that changes are being made is one thing, but responding in a timely manner is another issue.
Also, compliance in pharma organizations requires effective communication and collaboration among various departments, functions, and external partners. This can bring its own challenges, around data flow, sharing information, and visibility of processes.
Saurabh: What are some of the traditional methods that pharma companies have used to meet regulatory and compliance requirements?
Jane: Often, for large text-based projects such as updating drug labels, companies rely on manual methods for tracking and recording information and updates. Some still rely on Excel spreadsheets and Word documents, with manual copy-and-paste rather than automated or semi-automated processes. In addition, there is still a high reliance on employees’ implicit knowledge gained from on-the-job experience, and it can be hard to share best practices. These traditional manual text-based approaches can limit the ability of regulatory affairs professionals to respond in a timely manner without substantial time and effort.
Saurabh: How do manual-intensive processes in accessing key data hinder revenue growth for pharmaceutical companies?
Jane: Manual processes can be time-consuming, error-prone, and inconsistent, leading to delays, and rework across regulatory affairs, which can have a significant impact on revenue. For example, when submitting a drug approval package, there is a huge amount of manual effort, combining and reviewing content from the subsidiary documents to the master document set. If there are significant errors, drug regulators delay the approval, asking for amendments and corrections. These delays can be costly – a newly launched blockbuster drug could make $2bn in the first year, which is $6M per day. So, if errors hold up an application by even a week, this can have a serious impact on revenue.
While new drug approvals are relatively rare events, there are many regulatory processes that impact the bottom line. In a highly competitive industry, pharma companies that can navigate the regulatory landscape efficiently gain a competitive edge. Manual compliance processes can make it challenging to respond swiftly to changes in regulations or seize new market opportunities, putting the company at a disadvantage compared to more agile competitors.
Saurabh: What do you think are the benefits of changing document-driven processes to data-driven ones?
Jane: Transitioning to data-driven processes can yield many benefits. These include:
- Improved efficiency and time savings: Data-driven processes automate and streamline tasks that were previously manual and document-centric. Regulatory professionals can then spend less time searching for and organizing documents and more time analyzing and acting upon the information. This efficiency leads to faster submission preparation and other regulatory document creation and review, which in turn accelerates product launches and product management once on the market.
- Enhanced compliance and accuracy: Data-driven systems offer better control over compliance-related activities. Automated checks and validations ensure that regulatory documents are accurate, consistent, and aligned with regulatory standards. This reduces the risk of errors, discrepancies, and non-compliance, which could lead to costly delays, fines, or reputational damage.
- Informed decision-making: Data-driven processes provide access to real-time insights and analytics. Regulatory professionals can make informed decisions based on up-to-date data, historical trends, and predictive analytics. This empowers them to proactively address challenges, optimize strategies, and allocate resources effectively. Digitalizing, surfacing and analyzing the metadata around regulatory document authoring, for example, can bring insights into where there are bottlenecks, and enable teams to measure, and therefore improve, processes.
Saurabh: What role does natural language processing (NLP) play in addressing regulatory and compliance challenges for pharma companies?
Jane: To maintain compliance with constantly shifting regulations, pharmaceutical companies need tools that enable them to discover, highlight, and extract key data within regulatory documents. Many regulatory teams have employed NLP, an innovative artificial intelligence-based technology, to accomplish these goals.
One key attribute of NLP within drug development is the ability to surface information and insights, without having to manually read each document. NLP text mining uses AI-based technologies to transform the free, or unstructured, text in documents and databases into normalized, structured data suitable for analysis.
NLP enables pharmaceutical companies to examine large collections of documents to discover the information within the text and help answer specific research or regulatory questions. The process is useful for identifying facts, relationships, and assertions that would otherwise remain buried in huge volumes of textual data. After this information is converted into a structured form that can be further analyzed, integrated into databases, data warehouses, or business intelligence dashboards and used for descriptive, prescriptive, or predictive analytics.
Saurabh: Can you provide examples of specific ways in which pharma companies have successfully used NLP to address regulatory challenges?
Jane: We could provide many, but I’ll focus on a couple.
The first one is around drug labeling, and access to published reference labels that all pharma regulatory teams need. When developing content for new drug labels, life sciences companies’ regulatory teams face challenges in gathering intelligence to better understand competitive factors, market dynamics, and effective approval strategies. One top 10 pharma company turned to NLP to help. They used a drug label exploration tool that integrated NLP with key label sources (including FDA, EMA, UK, France and Spanish Drug Labels). NLP enabled users to search effectively, using linguistic and semantic searches, to answer their most important questions and find the data they needed. They could also select specific labels for comparison through an interactive view, with the NLP providing analysis and highlighting of the differences between labels. They found that using the label intelligence tool decreased the time taken for label comparison from 8 hours per label to 15 minutes – a 30-fold improvement. This application of NLP for label access and analysis saves the team time, making the process of developing new labels, updating existing labels, and securing regulatory approval faster and easier.
The second example concerns compliance with IDMP (IDentification of Medicinal Products). IDMP is a set of international standards, developed by the ISO, to define the rules that uniquely identify medicinal products and the relevant elements to identify them. But one of the key challenges is that many of the data elements required are buried in textual documents such as labels and dossiers. A top 10 pharma consumer division used NLP to find and extract over 140 IDMP data attributes from a range of regulatory documents including Summary of Product Characteristics and regulatory dossiers (eCTD sections 3.2.S and 3.2.P). The challenges are familiar to anyone involved in IDMP compliance - varied document sets, in mixed formats (Doc, Docx, image and text PDFs), across different languages, integrating with SPOR vocabularies. The output needed to be mapped to the regulatory MDM schema for their IDMP submission and internal business use. NLP was highly effective for data extraction and document processing, saving the team significant time and resources.
Saurabh: Are there any potential risks or limitations associated with the use of NLP in regulatory compliance for pharma companies?
Jane: NLP is a powerful technology that can help pharma companies improve their regulatory compliance activities by enabling more effective access and analysis of textual documents. However, like any technology, NLP also has potential risks that must be considered.
Access to data and documents is critical. Bringing NLP into regulatory compliance relies on the availability of the underlying document sources that it processes, and the accuracy of the data within these. If the data is incomplete, inaccurate, inconsistent, outdated, or inaccessible, the results of NLP may be unreliable or invalid.
Additionally, there is a range of NLP technologies, and pharma companies need to know which tool is appropriate for the different tasks in regulatory compliance. Rule-based technologies have been shown to be very effective at clean accurate information retrieval and extraction. There’s currently a lot of excitement around new technologies such as large language models, which can be hugely valuable for generative text, question-answering, and text summarization. However, there are risks around using technology for compliance that have not been fully tried and tested for that use, and these “black-box” machine learning algorithms may have inherent or learned biases that can affect their performance and outcomes.
Thus, while NLP can automate many tasks, human inspection and control is still necessary to ensure the accuracy and reliability of compliance-related decisions. Relying solely on NLP without human validation could lead to errors. Pharma companies may need to demonstrate how they ensure the validity and security of their data and algorithms. Within regulatory affairs, it’s better to think of AI as “augmented intelligence” and keep a human in the loop to provide oversight.
Image Source: Canva
About the Author:
Jane Reed
Jane Reed is the Director of Life Science at IQVIA NLP (formerly Linguamatics). She is responsible for leading the strategic vision for Linguamatics product portfolio and business development for the pharma and biotech market, with a focus on safety and regulatory affairs. Jane has 20+ years’ experience in vendor companies supplying data products, data integration and analysis, and consultancy to pharma and biotech—with roles at Instem, BioWisdom, Incyte, and Hexagen. Before moving into the life science industry, Jane worked in academia with post-docs in genetics and genomics. Jane holds an MA from Cambridge University and a PhD from Birmingham University.
Tags
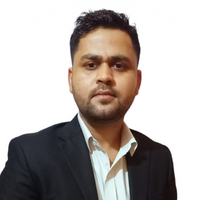
Saurabh is a Senior Content Writer at PharmaShots. He is a voracious reader and follows the recent trends and innovations of life science companies diligently. His work at PharmaShots involves writing articles, editing content, and proofreading drafts. He has a knack for writing content that covers the Biotech, MedTech, Pharmaceutical, and Healthcare sectors.